AI adoption in hospitals - Michael Page (Unity health)
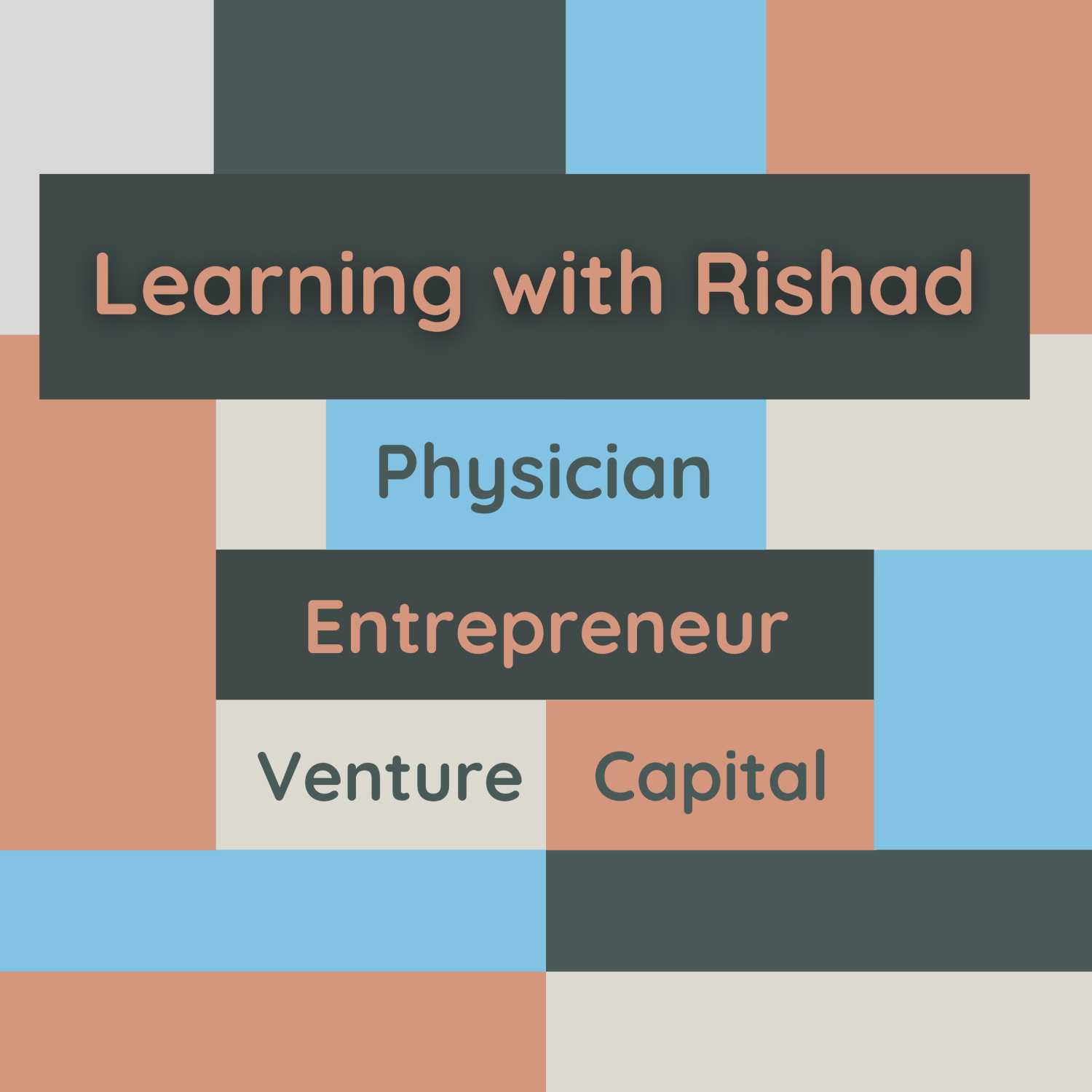
In this episode, I interview Michael Page about his fascinating and unconventional career path. Michael shares his story, from growing up in a working-class family and pursuing a degree in the arts, to transitioning into technology and AI, and now teaching at IV Business School and working in healthcare AI at Unity Health. We discuss the challenges and lessons learned along his journey, his insights on team building, the complexities of innovation in Canadian healthcare, his vision for advanced AI in medicine, and much more.
- (0:16) Michael's Childhood & Path: Michael talks about growing up in a lower-income, working-class family, being the first person in his immediate family to attend university, and how that experience shaped his journey.
- (2:32) Pursuing Arts at UFT: Michael shares how he ended up pursuing a Humanities degree at the University of Toronto, how theatre helped him find his voice, and the critical thinking skills he gained from his arts background.
- (5:42) Transition to Business and AI: Michael discusses his move from working in various arts organizations to the business side at UFT, and the key factors, including developing his management mindset and being exposed to cutting-edge AI research, that led him into technology and AI.
- (12:32) On "Hire Slow, Fire Fast": Michael offers his perspective on this common startup philosophy, emphasizing the importance of thoughtful hiring and reflecting on the time it takes for individuals to truly contribute effectively.
- (14:27) Avoiding Bad Hires: Michael shares his experiences with making bad hires and discusses the challenges and biases inherent in the traditional hiring process, suggesting ways to look beyond standard criteria to find committed individuals.
- (20:29) Thoughts on Remote Work & Culture: Michael talks about the benefits and challenges of remote and hybrid work and discusses how teams can actively build and maintain culture in both in-person and virtual environments, using examples like virtual coffee chats.
- (24:58) Changing Canadian Healthcare: If he had a magic wand, Michael says the one thing he would change about Canadian healthcare is removing the perceived antagonism between providers and patients, fostering a mindset of shared goals.
- (27:12) Building with Infinite Funds: Michael shares his vision for what he would build in healthcare with significant funding: a robust, multimodal AI company that can process various types of data to revolutionize patient care.
- (30:19) Addressing AI Errors and Drift: Michael discusses the phenomena of AI drift and how to manage for accuracy in AI tools, using the example of an emergency department volume prediction tool.
- (34:46) Should AI Be Explainable?: Michael gives his opinion on the mandate for AI explainability in healthcare, arguing that explainability is often mistakenly equated with trustworthiness and safety.
- (37:48) Why Hospitals Struggle with Innovation: Michael discusses the challenges hospitals face in being innovative, including top-down approaches and failing to include key stakeholders like nurses in the process.
- (39:43) Canada's Innovation Record: Michael shares statistics about Canada's low corporate R&D spending compared to other G8 countries across various industries.
- (41:01) The Problem with Governance: Michael highlights how overly long and expensive governance processes can stifle innovation in organizations.
- (43:25) Structural Issues in Healthcare & Academia: Michael discusses structural reasons why Canadian healthcare and academic institutions may lag in innovation, pointing out the lack of certain leadership roles.
- (45:21) Advice to His Younger Self: Michael shares the one piece of advice he would give himself 20 years ago.
1
00:00:00,000 --> 00:00:13,400
Hi Michael, it's great to have you with us today.
2
00:00:13,400 --> 00:00:14,400
Yeah, thanks for having me.
3
00:00:14,400 --> 00:00:16,040
It's wonderful to be here.
4
00:00:16,040 --> 00:00:21,280
If you could tell us a bit about your childhood and go as in-depth as you'd like to about
5
00:00:21,280 --> 00:00:23,480
the past to where you are now.
6
00:00:23,480 --> 00:00:25,480
Yeah.
7
00:00:25,480 --> 00:00:27,840
Where do you begin with childhood?
8
00:00:27,840 --> 00:00:34,960
I think maybe, you know, I tell some people this and maybe it's surprising, maybe it's
9
00:00:34,960 --> 00:00:39,120
not because maybe I like I present a certain way or maybe there's some assumptions of
10
00:00:39,120 --> 00:00:41,320
who I am.
11
00:00:41,320 --> 00:00:52,000
Grew up in sort of this like suburban countryside and sort of a lower income working class family.
12
00:00:52,000 --> 00:00:58,920
So I'd say childhood was really, really great filled with creativity and a lot of time outdoors
13
00:00:58,920 --> 00:01:04,760
and not a lot of time in technology, which I think some people sort of assume.
14
00:01:04,760 --> 00:01:09,040
I might be the kid that's like taking apart radios and try to figure out how to put them
15
00:01:09,040 --> 00:01:15,280
back together the same as like my bike or something else around the garage.
16
00:01:15,280 --> 00:01:22,480
So probably the most impressionable thing is being the first person in my immediate family
17
00:01:22,480 --> 00:01:25,240
to go to university.
18
00:01:25,240 --> 00:01:28,440
And so, you know, education was really important.
19
00:01:28,440 --> 00:01:34,640
It was hard to navigate that as being, you know, kind of that outsider.
20
00:01:34,640 --> 00:01:38,840
My parents kind of, you know, steering me as much as they could, but more things being
21
00:01:38,840 --> 00:01:42,160
unknown than known for them.
22
00:01:42,160 --> 00:01:47,040
And you know, that took me to an incredible path of, you know, everyone says like follow
23
00:01:47,040 --> 00:01:48,040
your passion.
24
00:01:48,040 --> 00:01:52,920
So I started my career in the arts and maybe we could talk a little bit about that.
25
00:01:52,920 --> 00:01:57,840
But where I am today is I ended up going back and doing a master's.
26
00:01:57,840 --> 00:02:02,360
I now work in technology and AI of all things.
27
00:02:02,360 --> 00:02:08,400
And for over a year now, I've been at the Ivy Business School at Western University
28
00:02:08,400 --> 00:02:09,400
now teaching.
29
00:02:09,400 --> 00:02:18,440
So my kind of like full circle moment is being this loved and supported working class kid
30
00:02:18,440 --> 00:02:24,560
to be the first person to go to university to now teach in a university, which I think
31
00:02:24,560 --> 00:02:30,080
is just, you know, if not through education, then it has to be Canada's the only place
32
00:02:30,080 --> 00:02:34,040
where you can make that happen is what I believe.
33
00:02:34,040 --> 00:02:35,040
That's amazing to hear.
34
00:02:35,040 --> 00:02:40,360
So let's go back to your time in high school.
35
00:02:40,360 --> 00:02:47,400
And how did you decide to do a bachelor's in arts and talk to me about your experience
36
00:02:47,400 --> 00:02:52,880
there and then your transition to working for the Toronto Symphony?
37
00:02:52,880 --> 00:02:53,880
Yeah.
38
00:02:53,880 --> 00:02:56,400
Great question.
39
00:02:56,400 --> 00:03:05,000
So I end up becoming sort of this like, you know, kind of now comfortable in crowds and
40
00:03:05,000 --> 00:03:08,920
can sort of speak my mind freely.
41
00:03:08,920 --> 00:03:12,400
But that's not where I started in high school.
42
00:03:12,400 --> 00:03:17,400
I think it was for a good period of time, you know, by sweating through my shirt afraid
43
00:03:17,400 --> 00:03:21,360
of my own shadow a little bit too much.
44
00:03:21,360 --> 00:03:28,120
But at some point in high school, I became kind of the theater kid.
45
00:03:28,120 --> 00:03:33,160
And through, you know, those classes, those opportunities, we had like an improv group
46
00:03:33,160 --> 00:03:35,200
and doing some plays.
47
00:03:35,200 --> 00:03:41,840
I kind of found my voice and being able to talk to people and relate to people and maybe
48
00:03:41,840 --> 00:03:48,440
also being more confident being another character rather than being myself is probably part of
49
00:03:48,440 --> 00:03:50,000
the story.
50
00:03:50,000 --> 00:03:56,440
And so when I was choosing universities, what's interesting is I applied in sort of like a
51
00:03:56,440 --> 00:03:58,360
really eclectic way.
52
00:03:58,360 --> 00:04:04,280
So on one hand, there was like fine arts and sort of bachelor arts programs.
53
00:04:04,280 --> 00:04:09,360
And then on the other hand, were business and technology programs.
54
00:04:09,360 --> 00:04:16,200
And I had actually committed accepted to a different university to do a business and
55
00:04:16,200 --> 00:04:17,200
technology.
56
00:04:17,200 --> 00:04:20,800
I think it was a B.com, but I don't remember.
57
00:04:20,800 --> 00:04:26,840
And on the last day when you can decide or would have you back then, well before email,
58
00:04:26,840 --> 00:04:35,640
I got the acceptance letter from the University of Toronto to do a humanities degree.
59
00:04:35,640 --> 00:04:41,360
And on the very last day, my girlfriend at the time was at the university and I decided
60
00:04:41,360 --> 00:04:48,240
to go to U of T and just sort of pursue the arts and pursue what I love.
61
00:04:48,240 --> 00:04:51,280
I am only able to say this in hindsight.
62
00:04:51,280 --> 00:04:54,720
I don't think I appreciate it at the time.
63
00:04:54,720 --> 00:04:56,760
And there's some brilliant people.
64
00:04:56,760 --> 00:05:02,760
Like I think this quote is loosely based on something that Hal Jackman has said, where
65
00:05:02,760 --> 00:05:07,760
humanities degree or the arts teaches you how to think critically.
66
00:05:07,760 --> 00:05:12,320
It's actually not really about analysis of art in and of itself.
67
00:05:12,320 --> 00:05:15,360
It's about how are you structuring thought?
68
00:05:15,360 --> 00:05:21,120
How can you understand what other people believe, think and value?
69
00:05:21,120 --> 00:05:22,920
And then how do you communicate that?
70
00:05:22,920 --> 00:05:27,080
So that was kind of my takeaway.
71
00:05:27,080 --> 00:05:30,720
And I found myself there by circumstance.
72
00:05:30,720 --> 00:05:37,320
I wish I was probably more intentional at the time, but I did walk away with some skills
73
00:05:37,320 --> 00:05:43,880
and some purpose and some this growth mindset of what I wanted to do in the world.
74
00:05:43,880 --> 00:05:49,400
And what prompted the transition to business and then to AI?
75
00:05:49,400 --> 00:05:51,000
That's a good point.
76
00:05:51,000 --> 00:05:57,360
So I started off at a few different arts organizations as you've noted.
77
00:05:57,360 --> 00:06:04,640
So I worked at a theater company in Branson, Ontario that was just opening up called the
78
00:06:04,640 --> 00:06:06,080
Rose Theater.
79
00:06:06,080 --> 00:06:11,600
I was there for the opening was like one of my first internships, bounced around at like,
80
00:06:11,600 --> 00:06:16,600
you know, a children's theater company, an arts service organization for playwrights.
81
00:06:16,600 --> 00:06:23,400
Found myself at the Toronto Symphony, where the symphony felt like the most professional
82
00:06:23,400 --> 00:06:25,360
place I had ever worked at that point.
83
00:06:25,360 --> 00:06:28,120
I'm like very early 20s.
84
00:06:28,120 --> 00:06:33,440
You know, they've got a board, a CEO, there's hundreds of people, there's lots of revenue
85
00:06:33,440 --> 00:06:34,720
flowing in.
86
00:06:34,720 --> 00:06:40,920
And this is the moment where I start to feel and understand and really experience all the
87
00:06:40,920 --> 00:06:44,840
different, you know, common nodes of business.
88
00:06:44,840 --> 00:06:52,240
So marketing and sales and HR and in our case, fundraising.
89
00:06:52,240 --> 00:06:57,560
And how do they function and how does sort of this corporate structure function?
90
00:06:57,560 --> 00:07:04,440
And, you know, I'll say I was good enough at fundraising and an open an opportunity to
91
00:07:04,440 --> 00:07:11,080
return to U of T where I would work as an administrator in their advancement office
92
00:07:11,080 --> 00:07:15,960
and and I'm spending a decade there grew a lot.
93
00:07:15,960 --> 00:07:20,280
I say it's like, you know, I feel like U of T's played a pivotal role where it's like,
94
00:07:20,280 --> 00:07:24,800
you know, your first university where you're becoming an adult and then kind of where like
95
00:07:24,800 --> 00:07:29,000
I actually grew up by by working there for a decade.
96
00:07:29,000 --> 00:07:38,440
But the transition from all of that into business and technology was very much related to, you
97
00:07:38,440 --> 00:07:42,160
know, first my like my management mindset at the time.
98
00:07:42,160 --> 00:07:48,320
And then the second thing, wow, what an incredible opportunity being at U of T talking about AI
99
00:07:48,320 --> 00:07:51,960
and you know, in the 2010s.
100
00:07:51,960 --> 00:07:57,640
So the first thing is my management mindset before I went back and did my MBA at Ivy Business
101
00:07:57,640 --> 00:08:02,800
School was I always kind of kept the mental note of bad managers and the things that they
102
00:08:02,800 --> 00:08:05,400
would do and we're kind of mentally commit to.
103
00:08:05,400 --> 00:08:09,080
I don't want to do this when I manage.
104
00:08:09,080 --> 00:08:12,520
And I kind of got to a point of like, you know, when you're leading fairly large teams
105
00:08:12,520 --> 00:08:18,600
or you know, really big revenue goals or projects or what have you where I didn't really have
106
00:08:18,600 --> 00:08:20,640
a great skill set, right?
107
00:08:20,640 --> 00:08:25,720
Like this skill set based on I should do things, you know, I should not do the things that
108
00:08:25,720 --> 00:08:30,120
I did not like is not really a strong management philosophy.
109
00:08:30,120 --> 00:08:34,800
And so I felt like, you know, this is a huge part of my career.
110
00:08:34,800 --> 00:08:35,880
I'm not done.
111
00:08:35,880 --> 00:08:42,520
I want to have 10x the impact that I've had thus far.
112
00:08:42,520 --> 00:08:48,000
And if there's if there's one thing I need to learn, I need to learn how to lead.
113
00:08:48,000 --> 00:08:56,360
So that's what took me to Ivy Business School and doing my executive MBA there and kind
114
00:08:56,360 --> 00:08:58,440
of learning and growing.
115
00:08:58,440 --> 00:09:03,560
The transition to technology happened at the same time and it had a lot to do with, you
116
00:09:03,560 --> 00:09:07,360
know, the people and the projects and things I was working at U of T.
117
00:09:07,360 --> 00:09:09,080
I'll just name job for a second.
118
00:09:09,080 --> 00:09:14,040
None of these individuals will remember who I am, but having the opportunity to get assigned
119
00:09:14,040 --> 00:09:20,200
to support or be on projects with some, you know, incredible researchers and then now like
120
00:09:20,200 --> 00:09:30,880
founders like Dr. Raquel Erdison, Dr. Jillian Hadfield and a few others where very early
121
00:09:30,880 --> 00:09:38,360
days like in the 2010s, I'm learning about autonomous vehicles and learning about that,
122
00:09:38,360 --> 00:09:40,600
you know, how did they actually learn?
123
00:09:40,600 --> 00:09:41,760
Why are they learning?
124
00:09:41,760 --> 00:09:46,960
Where is this going and kind of conceptualizing the future or that these systems could be really,
125
00:09:46,960 --> 00:09:55,560
really unsafe if they're not managed or we don't think about the ethics and equity is
126
00:09:55,560 --> 00:10:02,720
really what Dr. Hadfield has contributed to AI and our ecosystem.
127
00:10:02,720 --> 00:10:07,040
And so this planted the seed in me where it's like, you know, we're seeing tech everywhere.
128
00:10:07,040 --> 00:10:13,360
I feel like it's the largest growth industry in the world, but being at U of T in that
129
00:10:13,360 --> 00:10:20,840
moment around, you know, these descendants of Dr. Jeffrey Hinton and where U of T has
130
00:10:20,840 --> 00:10:23,520
kind of led the world.
131
00:10:23,520 --> 00:10:27,160
I had in my gut, but I was probably, you know, just surrounded by some incredible people
132
00:10:27,160 --> 00:10:31,200
where it's like AI is going to be really, really big.
133
00:10:31,200 --> 00:10:36,160
So the transition to business is, you know, MBA, this in depth kind of understanding of
134
00:10:36,160 --> 00:10:42,640
seeing research really at the bleeding edge at U of T and then saying, we got to do more
135
00:10:42,640 --> 00:10:45,040
than just publish papers.
136
00:10:45,040 --> 00:10:50,960
We don't need more citations collecting dust on a bookshelf somewhere.
137
00:10:50,960 --> 00:10:56,200
We have a chance as Canada to really own the podium here and let's figure out how we get
138
00:10:56,200 --> 00:11:05,280
this research out of our really amazing research institutions into companies into everyday life
139
00:11:05,280 --> 00:11:07,840
so that we can, you know, grow economically.
140
00:11:07,840 --> 00:11:14,080
We can create more jobs, but that we can contribute to the world in a meaningful way.
141
00:11:14,080 --> 00:11:20,960
So I joined the Vector Institute and that's been, you know, a wonderful organization that
142
00:11:20,960 --> 00:11:28,240
really grew and groomed me and taught me so much about machine learning.
143
00:11:28,240 --> 00:11:33,920
And now I find myself in healthcare where, you know, we're seeing this every single day
144
00:11:33,920 --> 00:11:37,960
in the way that our clinicians are treating patients.
145
00:11:37,960 --> 00:11:45,920
So along eclectic circus of a journey, but I do relate this a little bit back to, you
146
00:11:45,920 --> 00:11:52,360
think to that 17 or 18 year old humanities kid, a lot of this is based there of how can
147
00:11:52,360 --> 00:11:58,560
I understand what other people value or what they communicate and how can I think critically
148
00:11:58,560 --> 00:11:59,640
about these things.
149
00:11:59,640 --> 00:12:06,680
And AI for me is really about that of we have this massive corpus of information.
150
00:12:06,680 --> 00:12:07,680
How do you take it in?
151
00:12:07,680 --> 00:12:09,000
How do you think critically?
152
00:12:09,000 --> 00:12:14,360
How do you exercise judgment or build systems that are effective or safe?
153
00:12:14,360 --> 00:12:18,600
And so the eye rely on the business skills and, you know, and some of the hustle and
154
00:12:18,600 --> 00:12:21,160
acumen and stuff like that that I've built up.
155
00:12:21,160 --> 00:12:26,320
But a lot of this is that arts background of how do we think critically?
156
00:12:26,320 --> 00:12:30,600
How do we understand what the current context is?
157
00:12:30,600 --> 00:12:35,920
Does the common startup philosophy that says that you should hire slow and fire fast, do
158
00:12:35,920 --> 00:12:37,160
you agree with that?
159
00:12:37,160 --> 00:12:41,080
Oh, that's a great question.
160
00:12:41,080 --> 00:12:42,520
I like the higher slow.
161
00:12:42,520 --> 00:12:47,880
So like I think, you know, we're coming off a period where venture back companies got
162
00:12:47,880 --> 00:12:49,640
incredible valuations.
163
00:12:49,640 --> 00:12:54,720
They hired hundreds, sometimes thousands of people and really did not have the runway
164
00:12:54,720 --> 00:13:00,920
to sustainably support that head count.
165
00:13:00,920 --> 00:13:06,360
The fire fasting, I'd say like, you know, it depends on sort of the context.
166
00:13:06,360 --> 00:13:15,080
I don't know what the definition would be around that, but I find that most individuals
167
00:13:15,080 --> 00:13:16,680
are really learning a job.
168
00:13:16,680 --> 00:13:22,080
You're contributing, but you're learning a job probably for the first year.
169
00:13:22,080 --> 00:13:27,600
The second year is where, you know, you're moving beyond sort of this binary learning
170
00:13:27,600 --> 00:13:32,600
like, oh, I was giving a task, I executed the task.
171
00:13:32,600 --> 00:13:37,640
The second year is when you really understand that context, your internal context, and you
172
00:13:37,640 --> 00:13:42,880
understand the external context where you might be able to be a top contributor or not.
173
00:13:42,880 --> 00:13:48,040
Now, all it's that people don't show up, they're late or quality of work, all that, you know,
174
00:13:48,040 --> 00:13:55,280
aside, but I do take a longer term view, depending if that is a long term view for some people,
175
00:13:55,280 --> 00:14:00,720
on human capital in particular, because it organizations are weird.
176
00:14:00,720 --> 00:14:04,040
Everyone is unique and everyone says that they're unique.
177
00:14:04,040 --> 00:14:10,200
And I don't know that enough leaders reflect on how poor their internal contacts might be
178
00:14:10,200 --> 00:14:13,840
for individuals to succeed.
179
00:14:13,840 --> 00:14:17,560
And so I feel like it's around the year mark, sometimes it's shorter, sometimes it's longer,
180
00:14:17,560 --> 00:14:22,360
but I find that sort of that second year is when people really kind of hit their stride
181
00:14:22,360 --> 00:14:25,600
and you can really see the value that they bring.
182
00:14:25,600 --> 00:14:27,480
Have you ever made a bad hire?
183
00:14:27,480 --> 00:14:31,200
And is there something you could have done in the hiring process that would have stopped
184
00:14:31,200 --> 00:14:32,360
you from hiring them?
185
00:14:32,360 --> 00:14:39,520
Yeah, made a bad hire, for sure, multiple times, did not see it coming.
186
00:14:39,520 --> 00:14:45,920
So I think, you know, interviews are getting to be a little bit more interesting and better,
187
00:14:45,920 --> 00:14:54,120
but something that I really, really reflect on, this does, you know, follow the murder
188
00:14:54,120 --> 00:15:01,080
of George Floyd and Candace efforts and truth and reconciliation is, I do think that as leaders,
189
00:15:01,080 --> 00:15:06,720
managers, people hiring, we need to think about how fraught the hiring process is.
190
00:15:06,720 --> 00:15:12,040
And this has nothing to do with AI suggesting like who we should hire, what resumes we should
191
00:15:12,040 --> 00:15:20,360
read, the process has been fraught to hire the person that went to the best school, had
192
00:15:20,360 --> 00:15:25,800
the best network, had the best reference, it's sort of been coached to do the same.
193
00:15:25,800 --> 00:15:33,960
And I'll say like, you know, we've taken it in quote, a chance on a few people where the
194
00:15:33,960 --> 00:15:38,080
interview was clunky, or you know, there was something where we're like, oh, we're not
195
00:15:38,080 --> 00:15:46,760
too sure, you can't see hustle, you can't see commitment, you can't see drive, and
196
00:15:46,760 --> 00:15:51,840
you're 10 standardized questions, and the one or two assignments you give someone, or
197
00:15:51,840 --> 00:15:55,160
the, you know, one to three references that you check.
198
00:15:55,160 --> 00:16:03,800
So who a person is, you know, you might get that sometimes in an interview process.
199
00:16:03,800 --> 00:16:08,640
And that's something where you're building a relationship together, and that spark may
200
00:16:08,640 --> 00:16:09,640
or may not happen.
201
00:16:09,640 --> 00:16:16,160
But if you find someone that really is aligned with your personal purpose or your corporate
202
00:16:16,160 --> 00:16:22,160
purpose, and they will literally move mountains for you, that's a great hire.
203
00:16:22,160 --> 00:16:30,040
And you know, if they're a bit quirky or late or they've got too many cats, who cares?
204
00:16:30,040 --> 00:16:34,760
As long as they're kind of, you know, really devoted to where both of you can go together.
205
00:16:34,760 --> 00:16:38,400
Yeah, my kids would say you can never have too many cats.
206
00:16:38,400 --> 00:16:43,880
I know my son desperately wants cats and dogs.
207
00:16:43,880 --> 00:16:47,680
We had a dog, and we're now in the like in between phase.
208
00:16:47,680 --> 00:16:52,640
But yes, if we had 16 cats, he would love it.
209
00:16:52,640 --> 00:16:56,120
I think people underscore the importance of references.
210
00:16:56,120 --> 00:17:02,400
And there's a couple of ways to do it, I usually seek references from previous jobs that the
211
00:17:02,400 --> 00:17:07,000
founders don't provide readily, and I let them know that.
212
00:17:07,000 --> 00:17:11,240
Or you can ask for 10 references and say you will randomly call three of them.
213
00:17:11,240 --> 00:17:14,000
Now that's a big ask.
214
00:17:14,000 --> 00:17:18,840
And the job offer or the investment has to reflect that.
215
00:17:18,840 --> 00:17:24,680
But you want references where the founder has had conflict as well.
216
00:17:24,680 --> 00:17:30,160
I think so, and you know, and understanding how people navigate conflict, like sometimes
217
00:17:30,160 --> 00:17:32,560
it really shuts someone down.
218
00:17:32,560 --> 00:17:38,400
And other times, someone can take it on the chin and say, you know, thank you.
219
00:17:38,400 --> 00:17:39,400
I disagree.
220
00:17:39,400 --> 00:17:43,040
But I'm going to think about this or I'm going to learn from this, this opportunity.
221
00:17:43,040 --> 00:17:49,840
So I like how you how you navigate that, I think is, is, is quite important.
222
00:17:49,840 --> 00:17:56,560
I think like, you know, I've seen things where like some companies are doing like mixers or
223
00:17:56,560 --> 00:18:03,360
actually the hiring team is kind of not involved for a certain, you know, round or phase.
224
00:18:03,360 --> 00:18:10,120
It's someone on the team is like messages as a person, hey, are you free for coffee?
225
00:18:10,120 --> 00:18:11,440
That's what I'm interested in.
226
00:18:11,440 --> 00:18:16,400
You know, if this person for an hour or two hours or as coach, the references can put
227
00:18:16,400 --> 00:18:20,200
something together for me, that's fine.
228
00:18:20,200 --> 00:18:28,840
But you know, Karen and Kayla, who you're going to have to work with every single day.
229
00:18:28,840 --> 00:18:29,840
Can you get along?
230
00:18:29,840 --> 00:18:35,520
Like is there some sort of animosity or friction or is there actually, you know, some, some
231
00:18:35,520 --> 00:18:38,160
real bond here for, for whatever reason?
232
00:18:38,160 --> 00:18:42,960
Is there sort of that that spark of opportunity?
233
00:18:42,960 --> 00:18:48,320
What is hard, and I do feel like there's some people talking about this, but what is really,
234
00:18:48,320 --> 00:18:59,480
really hard in AI and in tech is we just have many, many engineers and computer scientists
235
00:18:59,480 --> 00:19:01,400
that are introverted.
236
00:19:01,400 --> 00:19:09,360
And so if you look at the traditional scorecard of, you know, character or leadership, education
237
00:19:09,360 --> 00:19:13,880
experience, whatever, like how we're going to score someone, that individual just might
238
00:19:13,880 --> 00:19:20,160
be really, really nervous to talk to you or to share in that way.
239
00:19:20,160 --> 00:19:24,440
So part of like, you know, do we need to rethink this as some of this just like, would you
240
00:19:24,440 --> 00:19:28,440
be open like we just text over the next like five days of like, you know, I'd love to hear
241
00:19:28,440 --> 00:19:34,440
your thoughts on this big announcement from, from the video, like what do you think the
242
00:19:34,440 --> 00:19:42,280
impact would be on, on our work or health Canada just issued updated regulation on AI's medical
243
00:19:42,280 --> 00:19:46,440
device like what do you thought we can ask that and you know, maybe I've been coached
244
00:19:46,440 --> 00:19:51,080
and trained and have more experience where off the cuff, I'm going to give you something
245
00:19:51,080 --> 00:19:56,880
that's that's eloquent, but maybe for that interview to introverted engineer or computer
246
00:19:56,880 --> 00:20:01,400
scientists, let's just do that over email or do that over Slack or do that over text
247
00:20:01,400 --> 00:20:03,160
messages or something else.
248
00:20:03,160 --> 00:20:07,040
And maybe that's the way that we're going to build trust in that relationship.
249
00:20:07,040 --> 00:20:09,240
Maybe that's the way that I should be evaluating you.
250
00:20:09,240 --> 00:20:15,400
So that's a long way of me sort of saying that like, the world has changed, you know,
251
00:20:15,400 --> 00:20:22,920
like we're working remotely, very, very often, have our hiring practices changed as as radically
252
00:20:22,920 --> 00:20:24,200
probably not.
253
00:20:24,200 --> 00:20:28,040
And we probably need to rethink it.
254
00:20:28,040 --> 00:20:29,800
What are your thoughts in remote work?
255
00:20:29,800 --> 00:20:37,920
And you have a similar culture remotely, because a lot of us, a lot of companies are
256
00:20:37,920 --> 00:20:41,720
mandating back in office.
257
00:20:41,720 --> 00:20:44,680
Where do you fall on that spectrum?
258
00:20:44,680 --> 00:20:50,640
So it's like a working parent hybrid is an incredible benefit.
259
00:20:50,640 --> 00:20:55,640
And I can't say that it's that it's not you mentioned you have kids.
260
00:20:55,640 --> 00:20:56,640
They're sick.
261
00:20:56,640 --> 00:20:59,840
All these crazy things that happen with with kids schedules.
262
00:20:59,840 --> 00:21:05,080
I know a lot of us also have like elder care responsibilities.
263
00:21:05,080 --> 00:21:11,480
So it makes it challenging to be in office and present as much as maybe you would like
264
00:21:11,480 --> 00:21:12,480
to be.
265
00:21:12,480 --> 00:21:15,760
There's the there's the old adage, right?
266
00:21:15,760 --> 00:21:17,440
Culture eats strategy for breakfast.
267
00:21:17,440 --> 00:21:22,880
So are we really fixated on our corporate strategy?
268
00:21:22,880 --> 00:21:26,120
Or are we really fixated on our corporate culture?
269
00:21:26,120 --> 00:21:29,080
And I think you can build this remotely.
270
00:21:29,080 --> 00:21:31,640
It is hard.
271
00:21:31,640 --> 00:21:34,000
It might actually be more expensive than what people think.
272
00:21:34,000 --> 00:21:39,280
Like I hope that founders are not choosing hybrid or remote because it's the cheap thing
273
00:21:39,280 --> 00:21:45,840
to do, but that it actually does resonate with the team or individuals or what it is
274
00:21:45,840 --> 00:21:51,840
that that you need to build or why you're building.
275
00:21:51,840 --> 00:21:58,560
You get a lot more accomplished in person, so I do see sort of, you know, community building
276
00:21:58,560 --> 00:22:05,000
this kind of like this culture building happens just a little bit more seamlessly and in person
277
00:22:05,000 --> 00:22:10,080
than it does virtually, but it doesn't mean that it's impossible.
278
00:22:10,080 --> 00:22:16,400
On my experience and kind of our team right now is you have to put effort on both sides.
279
00:22:16,400 --> 00:22:23,440
So we are hybrid, but we have a lot of people that are committed to like, when are we all
280
00:22:23,440 --> 00:22:28,240
going skating in a few weeks together as a team over lunch?
281
00:22:28,240 --> 00:22:34,400
This isn't dictated by the leadership team to say like, you know, 28th of the month is
282
00:22:34,400 --> 00:22:38,880
you know, skating day and we expect you like, no, like it's a real group of friends and
283
00:22:38,880 --> 00:22:43,040
community within the team saying there's literally a skating rink across the street.
284
00:22:43,040 --> 00:22:44,720
Who wants to go on this day?
285
00:22:44,720 --> 00:22:45,720
Yes.
286
00:22:45,720 --> 00:22:47,760
Do this as a team activity.
287
00:22:47,760 --> 00:22:55,080
So there's real effort on that side to build relationships, build trust, build friendships,
288
00:22:55,080 --> 00:23:01,720
get to know one another beyond the code or the deliverable or the tactic that you're
289
00:23:01,720 --> 00:23:02,960
working on.
290
00:23:02,960 --> 00:23:06,800
In the same time, you know, we've also grown remotely.
291
00:23:06,800 --> 00:23:13,840
We have people that are not working within 30 minutes or an hour of downtown Toronto.
292
00:23:13,840 --> 00:23:20,120
How do we maintain that same friendship bond culture virtually?
293
00:23:20,120 --> 00:23:23,520
So you know, there's there's stuff that randomly pops up.
294
00:23:23,520 --> 00:23:27,800
You got to be creative with how you're using Slack.
295
00:23:27,800 --> 00:23:35,440
And I'd say the team does a fairly good job of it, but you need to put effort in into
296
00:23:35,440 --> 00:23:37,360
both sides for sure.
297
00:23:37,360 --> 00:23:41,720
It's something I will actually just share about our team.
298
00:23:41,720 --> 00:23:46,800
We I don't actually know where the name came from, but for whatever reason, on a monthly
299
00:23:46,800 --> 00:23:53,480
basis, they created just a really quick internal application called Timbits.
300
00:23:53,480 --> 00:23:57,280
I don't want any kind of issues with the Tim Horns Corporation.
301
00:23:57,280 --> 00:23:58,280
It's spelled differently.
302
00:23:58,280 --> 00:24:00,480
It just sounds the same.
303
00:24:00,480 --> 00:24:05,880
But on a monthly basis, we just get an email of like, Hey, Mike, you're going to have coffee,
304
00:24:05,880 --> 00:24:11,440
your virtual coffee with Rashad, and you're not allowed to talk about work.
305
00:24:11,440 --> 00:24:14,160
There's some suggestions of things that you could talk about.
306
00:24:14,160 --> 00:24:17,880
And it randomly kind of assigns people on every month.
307
00:24:17,880 --> 00:24:20,360
So this isn't leadership being dictated.
308
00:24:20,360 --> 00:24:23,120
This isn't, you know, the standing meeting.
309
00:24:23,120 --> 00:24:29,400
This isn't, but it does fork force us to break the mold to say, you know what, I haven't
310
00:24:29,400 --> 00:24:33,640
talked to this ETL developer in six months.
311
00:24:33,640 --> 00:24:35,280
We never cross paths.
312
00:24:35,280 --> 00:24:39,640
They're not on, you know, my immediate team.
313
00:24:39,640 --> 00:24:40,640
This is great.
314
00:24:40,640 --> 00:24:41,640
What are you doing as a person?
315
00:24:41,640 --> 00:24:44,920
Did you enjoy the holidays or, you know, how are things going?
316
00:24:44,920 --> 00:24:51,880
So we're using tech and investing sort of both sides of like in person and virtual to
317
00:24:51,880 --> 00:24:55,160
really maintain our team culture.
318
00:24:55,160 --> 00:24:59,600
If you could wave a magic wand and change one thing about Canadian healthcare, what
319
00:24:59,600 --> 00:25:04,760
would you change?
320
00:25:04,760 --> 00:25:13,000
Working in healthcare at times, it feels like, it feels sometimes a little bit like everyone's
321
00:25:13,000 --> 00:25:14,000
at odds.
322
00:25:14,000 --> 00:25:20,280
So, you know, we read a lot of the media and it feels like it's sort of accusatory of
323
00:25:20,280 --> 00:25:25,000
physicians or providers are so slow or something like wait times are egregious because they're
324
00:25:25,000 --> 00:25:26,000
so slow.
325
00:25:26,000 --> 00:25:34,040
If I could wave a magic wand, the one thing I would change is I hope that we come to understanding
326
00:25:34,040 --> 00:25:41,840
that providers and patients actually want the same thing.
327
00:25:41,840 --> 00:25:46,880
We want to take care of our patients and as a patient, you know, I want to get the best
328
00:25:46,880 --> 00:25:50,720
care possible.
329
00:25:50,720 --> 00:25:55,400
What probably your average person doesn't know is all the constraints that are in the
330
00:25:55,400 --> 00:26:02,800
way to deliver, you know, max amount of coverage or the type of quality of care that all of
331
00:26:02,800 --> 00:26:04,280
us want.
332
00:26:04,280 --> 00:26:11,080
And a lot of this is regulatory, a lot of this is data access.
333
00:26:11,080 --> 00:26:13,560
Some of it is human capital.
334
00:26:13,560 --> 00:26:17,880
So I'd hope that I can't change all those things with this wand wand, but the one thing
335
00:26:17,880 --> 00:26:24,040
I would change is just that current, what I feel mindset of that we're at odds like
336
00:26:24,040 --> 00:26:28,760
providers and patients are sort of antagonistic with one another.
337
00:26:28,760 --> 00:26:32,840
I don't feel like it's real and I'd love to wave a wand and just remove that.
338
00:26:32,840 --> 00:26:37,560
Or we all are like, oh yeah, no, they actually want to take care of me and they chose this
339
00:26:37,560 --> 00:26:43,000
profession or you know, you know what, it's fair that this person's really frustrated.
340
00:26:43,000 --> 00:26:47,720
They couldn't get into my family practice for three weeks or four weeks because I'm so
341
00:26:47,720 --> 00:26:52,600
overbooked and now they have pneumonia or they just sat in my emergency department for
342
00:26:52,600 --> 00:26:55,760
12 or 16 hours before they saw me.
343
00:26:55,760 --> 00:26:57,920
And of course, they're really, really frustrated.
344
00:26:57,920 --> 00:27:04,640
So I'd love to sort of remove the animosity so that we could really build back a system
345
00:27:04,640 --> 00:27:07,720
that is reflective of who we are.
346
00:27:07,720 --> 00:27:15,800
If Sequoia or A16Z or General Catalyst sent you an email tomorrow said, Michael, here's
347
00:27:15,800 --> 00:27:17,480
$50 million.
348
00:27:17,480 --> 00:27:19,360
We want you to build something in healthcare.
349
00:27:19,360 --> 00:27:20,360
What would you build?
350
00:27:20,360 --> 00:27:21,360
All right, great.
351
00:27:21,360 --> 00:27:26,640
Well, 50 is not enough money if you're A16Z listening to this.
352
00:27:26,640 --> 00:27:29,720
$500 million, whatever the number is.
353
00:27:29,720 --> 00:27:35,040
So I think, you know, we are starting to see this.
354
00:27:35,040 --> 00:27:39,880
We see some of the potential and the type of quality of data that the Nordic countries
355
00:27:39,880 --> 00:27:41,440
have captured.
356
00:27:41,440 --> 00:27:49,840
And where we're really excited at Unity Health and St. Mike's is yes, AI is everywhere and
357
00:27:49,840 --> 00:27:51,240
this is another AI talk.
358
00:27:51,240 --> 00:27:52,240
I get it.
359
00:27:52,240 --> 00:27:53,920
We're done with that.
360
00:27:53,920 --> 00:27:55,520
We are just at the beginning.
361
00:27:55,520 --> 00:27:59,960
If we're just seeing the tip of the iceberg, we don't see what's below the water.
362
00:27:59,960 --> 00:28:10,160
And the thing that I would build is a real robust multimodal AI company.
363
00:28:10,160 --> 00:28:15,240
Our leader, my boss, Dr. Muhammad Nani, has been talking about this for years.
364
00:28:15,240 --> 00:28:22,120
But imagine a system that can not only read, which is what we have now, right?
365
00:28:22,120 --> 00:28:28,760
A lot of the systems are based on reading text data out of your electronic medical record.
366
00:28:28,760 --> 00:28:36,160
Imagine a future AI that can not only read, but can see because we've built in video feeds.
367
00:28:36,160 --> 00:28:40,200
Imagine a future AI system that can read, see, and hear because we're using auditory
368
00:28:40,200 --> 00:28:46,240
inputs from our emergency department or our surgical bays.
369
00:28:46,240 --> 00:28:51,080
Imagine that it's a system that can feel because it's getting sensor feedback or instrument
370
00:28:51,080 --> 00:28:55,720
feedback from any of the diagnostic machines we're using or any of the surgical devices
371
00:28:55,720 --> 00:28:58,240
that we're using.
372
00:28:58,240 --> 00:29:04,840
That's the type of AI that really feels science fiction and far-fetched, but where I'm getting
373
00:29:04,840 --> 00:29:12,760
excited because we are seeing things like Alpha Fold and the team getting a Nobel Prize
374
00:29:12,760 --> 00:29:15,200
just a few weeks ago.
375
00:29:15,200 --> 00:29:22,680
And that is really, yes, a big, massive data set and a huge feat, but we've really not
376
00:29:22,680 --> 00:29:27,040
tapped into all the data possible.
377
00:29:27,040 --> 00:29:34,800
And if we can get a team like the next DeepMind access to that type of information, and I
378
00:29:34,800 --> 00:29:39,520
think you would be aligned with this, imagine that we can start helping people before they
379
00:29:39,520 --> 00:29:46,800
arrive in clinic that you knew, based on your aura ring and your feedback, I got to actually
380
00:29:46,800 --> 00:29:52,760
get on medication or I need to go to St. Mike's because this is actually pretty severe.
381
00:29:52,760 --> 00:29:57,520
And so the thing I would build is something around multimodal AI.
382
00:29:57,520 --> 00:30:03,080
And I think it's bigger than one medical discipline, one clinical area.
383
00:30:03,080 --> 00:30:07,720
I think it's widespread because medicine is interdisciplinary.
384
00:30:07,720 --> 00:30:14,760
It's going to take in data and data types and feeds from across the care spectrum.
385
00:30:14,760 --> 00:30:21,240
You know, lately we've been hearing that AI, in some circles that AI is getting dumber,
386
00:30:21,240 --> 00:30:26,760
and which is attributed to the phenomenon called drift from what I understand AI just
387
00:30:26,760 --> 00:30:28,840
goes wonky.
388
00:30:28,840 --> 00:30:30,280
How do you control for that?
389
00:30:30,280 --> 00:30:32,160
How do you fix that?
390
00:30:32,160 --> 00:30:44,720
Yeah, I think we are seeing that drift is not a guarantee from at least my reading and
391
00:30:44,720 --> 00:30:45,720
understanding.
392
00:30:45,720 --> 00:30:47,520
So it may or may not occur.
393
00:30:47,520 --> 00:30:52,200
And that's sort of the interesting phenomena to me of like, why is this or when is this
394
00:30:52,200 --> 00:30:55,880
not happening?
395
00:30:55,880 --> 00:31:03,120
Some of our models which are not LMS are just the R machine learning and some rules based
396
00:31:03,120 --> 00:31:05,000
systems, they're not getting dumber.
397
00:31:05,000 --> 00:31:07,440
The accuracy is staying the same.
398
00:31:07,440 --> 00:31:14,600
But the part that I think maybe we need to keep in mind is our current context does change.
399
00:31:14,600 --> 00:31:16,240
So I'll give you an example.
400
00:31:16,240 --> 00:31:21,040
We've got a tool that predicts volumes into our emergency department at St. Mike's and
401
00:31:21,040 --> 00:31:22,040
St. Joe's.
402
00:31:22,040 --> 00:31:28,640
It's 48 to 72 hours in advance and it's very, very accurate, over 90% accurate, I think
403
00:31:28,640 --> 00:31:30,760
over 95% accurate.
404
00:31:30,760 --> 00:31:37,840
The part that we've done that's different than some other areas is we're able to predict
405
00:31:37,840 --> 00:31:41,280
severity of cases and some key areas for us.
406
00:31:41,280 --> 00:31:49,400
So we'll know, okay, you know, on Monday morning, we are going to have 30 cases, 28 of them are
407
00:31:49,400 --> 00:31:53,760
going to be pretty easy, two of them are going to be difficult and five of them are going
408
00:31:53,760 --> 00:31:55,840
to be mental health cases.
409
00:31:55,840 --> 00:32:00,880
What is this tool trained on or where is it getting its data from just in general?
410
00:32:00,880 --> 00:32:06,200
Yeah, so this is, it's a time series model.
411
00:32:06,200 --> 00:32:11,680
And so we're doing inputs of sort of our historical data at our emergency departments of the types
412
00:32:11,680 --> 00:32:12,680
of visits.
413
00:32:12,680 --> 00:32:17,400
And there's a lot of literature, I think going back to the 70s of that, emergency department
414
00:32:17,400 --> 00:32:21,720
visits are very predictable and there's seasonality associated with it.
415
00:32:21,720 --> 00:32:27,480
As Canadians, we should understand that there's cold and flu season for, for a reason.
416
00:32:27,480 --> 00:32:30,520
For our hospital too, there's also trauma care season.
417
00:32:30,520 --> 00:32:36,620
So please wear your helmets when you're riding your bike or going skating.
418
00:32:36,620 --> 00:32:40,640
So we see these natural spikes and occurrences and they do repeat themselves on an annual
419
00:32:40,640 --> 00:32:41,900
basis.
420
00:32:41,900 --> 00:32:51,880
The other key input for us too, actually, is we input weather and we input cultural events
421
00:32:51,880 --> 00:32:53,520
in the city.
422
00:32:53,520 --> 00:32:58,200
So really, really high likelihood, but we know, you know, if there's a hockey game and
423
00:32:58,200 --> 00:33:03,320
freezing rain and it's a Saturday night during cold and flu season, it's probably going to
424
00:33:03,320 --> 00:33:05,480
be a bad night in our emergency department.
425
00:33:05,480 --> 00:33:11,080
And when you say you input cultural events, like what is actually happening?
426
00:33:11,080 --> 00:33:17,160
Yeah, so we have basically like a listening type bot that there's a few different sites
427
00:33:17,160 --> 00:33:19,640
that's looking for posted dates.
428
00:33:19,640 --> 00:33:25,680
So hockey games is, it's one of the big ones basketball games.
429
00:33:25,680 --> 00:33:30,640
We you know, if there's parades and stuff like that, there's just more slips, trips,
430
00:33:30,640 --> 00:33:32,000
bumps and falls.
431
00:33:32,000 --> 00:33:34,120
And so therefore, where do they arrive?
432
00:33:34,120 --> 00:33:36,480
They arrive at downtown hospitals.
433
00:33:36,480 --> 00:33:42,280
And it's able to kind of say a hockey game will have one extra patient, whereas a Taylor
434
00:33:42,280 --> 00:33:45,480
Swift concert might have 100 extra patients or.
435
00:33:45,480 --> 00:33:52,360
Yeah, I don't know the volumes and how that, how those two sort of things equate.
436
00:33:52,360 --> 00:33:54,720
I will tell you the thing that we missed.
437
00:33:54,720 --> 00:34:00,160
I don't know if the listening tool didn't have this, but the thing where we had many
438
00:34:00,160 --> 00:34:05,360
more people in the emergency department than what we were expecting was when our world
439
00:34:05,360 --> 00:34:10,160
championship Toronto Raptors had their parade.
440
00:34:10,160 --> 00:34:16,520
And we, you know, I don't think the city was ready for that celebration.
441
00:34:16,520 --> 00:34:20,720
But the emergency department, it was like we weren't ready, but we saw many more people
442
00:34:20,720 --> 00:34:24,080
than what we were anticipating that day.
443
00:34:24,080 --> 00:34:26,680
So that's one of the, one of the misses.
444
00:34:26,680 --> 00:34:33,240
But the, yeah, I don't know that we take into account the volume of the event, but just
445
00:34:33,240 --> 00:34:38,680
certain events like, you know, marathons and stuff like that, the people do get hurt and
446
00:34:38,680 --> 00:34:42,480
they do end up in our emergency department.
447
00:34:42,480 --> 00:34:48,360
Do you think we should mandate explainability for AI as some of our medical societies have
448
00:34:48,360 --> 00:34:54,160
come out with and said, you know, if you're using an AI, you need to understand at some
449
00:34:54,160 --> 00:35:00,440
level how it works, but AI, you know, is a black box for the most part.
450
00:35:00,440 --> 00:35:01,440
Yeah.
451
00:35:01,440 --> 00:35:07,920
So I think explainability has caught on somewhat unfortunately.
452
00:35:07,920 --> 00:35:11,360
And so there's a few things to be the backup and say about this.
453
00:35:11,360 --> 00:35:15,160
So no, I don't think that we should mandate explainability and feel like also that we've
454
00:35:15,160 --> 00:35:16,680
solved the problem.
455
00:35:16,680 --> 00:35:23,720
I feel like explainability is caught on as a surrogate for trust and safety.
456
00:35:23,720 --> 00:35:29,080
So if we can understand something is kind of the argument, oh, then therefore it is trustworthy
457
00:35:29,080 --> 00:35:31,000
or then therefore it is safe.
458
00:35:31,000 --> 00:35:32,000
And that's actually not true.
459
00:35:32,000 --> 00:35:36,920
You can understand something and it could still not be trustworthy and it could still
460
00:35:36,920 --> 00:35:38,440
not be safe.
461
00:35:38,440 --> 00:35:43,360
The other example, you know, I've heard many people sort of say, you know, there's actually
462
00:35:43,360 --> 00:35:53,120
a lot of really common medications that all of us use regularly that we don't understand
463
00:35:53,120 --> 00:35:55,480
that we can't explain.
464
00:35:55,480 --> 00:35:59,360
You can ask your average physician or nurse, what is the mechanism of action for a CETA
465
00:35:59,360 --> 00:36:02,680
medifin and they're probably going to get it wrong.
466
00:36:02,680 --> 00:36:08,760
But yet it's, you know, one of the most widely used pharmaceuticals in the world.
467
00:36:08,760 --> 00:36:13,280
We all drive cars or get on trains and buses, but we don't necessarily understand, you know,
468
00:36:13,280 --> 00:36:17,880
how does a V8 or V12 engine works anymore?
469
00:36:17,880 --> 00:36:21,240
What is the difference between combustion or electric engines?
470
00:36:21,240 --> 00:36:26,520
So I don't think that explainability takes us to the place that we want to go.
471
00:36:26,520 --> 00:36:30,320
I think it just sort of caught on of like, oh, you know, if I understand more, I'm going
472
00:36:30,320 --> 00:36:32,880
to feel safer.
473
00:36:32,880 --> 00:36:37,760
And the reality with AI is you might not understand something, but still it might be really, really
474
00:36:37,760 --> 00:36:39,280
unsafe.
475
00:36:39,280 --> 00:36:47,600
So the part where I'm interested is what are the monitors and evaluations that are in place.
476
00:36:47,600 --> 00:36:50,320
And I don't know how we necessarily regulate that.
477
00:36:50,320 --> 00:36:52,640
That's not my space to dictate.
478
00:36:52,640 --> 00:36:59,800
But what I would want to see is for critical systems that have an impact in hurting someone,
479
00:36:59,800 --> 00:37:05,560
that they're monitored, and that there's some type of regular evaluation in place.
480
00:37:05,560 --> 00:37:11,520
That's what would make me feel safer and that we're in a better spot.
481
00:37:11,520 --> 00:37:19,120
Throughout history, we have been the smartest being on earth as far as we know.
482
00:37:19,120 --> 00:37:24,560
And now we're creating something which is more creative and smarter than us.
483
00:37:24,560 --> 00:37:26,880
And I think this scares us.
484
00:37:26,880 --> 00:37:34,040
And I think this is where this rise of explainability comes from is, okay, if I can explain it,
485
00:37:34,040 --> 00:37:36,600
I'm smarter than it.
486
00:37:36,600 --> 00:37:43,720
And I think that that's what's driving these rules and regulations.
487
00:37:43,720 --> 00:37:50,160
You sit at an intersection of industry and healthcare.
488
00:37:50,160 --> 00:37:59,800
What advice would you give to hospitals who are looking to be more innovative and incorporate
489
00:37:59,800 --> 00:38:03,840
more AI and startups into their ecosystem?
490
00:38:03,840 --> 00:38:10,160
And what are some things they do wrong?
491
00:38:10,160 --> 00:38:18,480
So I think it really comes down to identifying the problem and picking the right problem.
492
00:38:18,480 --> 00:38:24,360
And there's many different ways that you can go about that.
493
00:38:24,360 --> 00:38:28,560
What I see, whether you're building or you're buying something, like you're going to partner
494
00:38:28,560 --> 00:38:34,560
with a startup or what have you, innovation is this big, sexy thing.
495
00:38:34,560 --> 00:38:39,600
And a lot of people on the leadership team get involved in these meetings and issue the
496
00:38:39,600 --> 00:38:41,800
RFP, what have you.
497
00:38:41,800 --> 00:38:47,680
And I'll just be honest, I don't know many nurses directly.
498
00:38:47,680 --> 00:38:52,160
I'm not funded or supported by any sort of nursing union or federation.
499
00:38:52,160 --> 00:38:58,360
But you go through these processes and typically nurses are not included whatsoever.
500
00:38:58,360 --> 00:39:01,360
And it's such a huge miss for acute care.
501
00:39:01,360 --> 00:39:07,040
I can't speak to clinical practice specifically.
502
00:39:07,040 --> 00:39:12,800
But for acute care, where it's like something 80% or like 70% of bedside care is delivered
503
00:39:12,800 --> 00:39:16,520
by nurses, but they're not part of the innovation RFP process.
504
00:39:16,520 --> 00:39:22,760
Or they don't have a mechanism to share their challenges or pain points.
505
00:39:22,760 --> 00:39:30,600
So that for me is like a big miss of not including everyone within that practice as part of the
506
00:39:30,600 --> 00:39:36,880
journey, identifying the right end users as well.
507
00:39:36,880 --> 00:39:43,400
So we're still seeing very much a top down model.
508
00:39:43,400 --> 00:39:50,160
And I think we need to be honest, our innovation record in healthcare, but across industries
509
00:39:50,160 --> 00:39:54,220
in Canada is one of the worst in the world.
510
00:39:54,220 --> 00:40:02,320
So we have the lowest corporate R&D expenditure on technology in the G8.
511
00:40:02,320 --> 00:40:06,280
So we find ourselves below Italy.
512
00:40:06,280 --> 00:40:10,160
So that's not something that we might kind of like really conceptualize and think about.
513
00:40:10,160 --> 00:40:17,200
This isn't healthcare being less than average at its ability to innovate.
514
00:40:17,200 --> 00:40:23,160
We're seeing this in financial services, in manufacturing, in forestry, in education,
515
00:40:23,160 --> 00:40:28,520
in any industry in Canada, we find ourselves in the last place of the G8.
516
00:40:28,520 --> 00:40:33,920
So there's kind of this thing, like if you do something wrong once, do you repeat that
517
00:40:33,920 --> 00:40:35,960
pattern or do you actually change it?
518
00:40:35,960 --> 00:40:41,640
We find ourselves decades into this journey of we keep repeating the same pattern.
519
00:40:41,640 --> 00:40:44,880
And what I would say is we need to break the model.
520
00:40:44,880 --> 00:40:46,280
We need to try new things.
521
00:40:46,280 --> 00:40:53,600
Probably the same model doesn't work for each organization or each individual, but not including
522
00:40:53,600 --> 00:40:59,800
your people in that process, in that journey is a huge, huge miss.
523
00:40:59,800 --> 00:41:07,520
My second thing that probably we'll get some hate mail for this one, but I've said it before,
524
00:41:07,520 --> 00:41:09,120
so it's okay.
525
00:41:09,120 --> 00:41:19,280
The second fallacy that I think organizations fall into is a very long, protracted, expensive
526
00:41:19,280 --> 00:41:21,280
governance process.
527
00:41:21,280 --> 00:41:27,080
Okay, so we are going to innovate XYZ.
528
00:41:27,080 --> 00:41:33,320
So we need to talk to privacy, we need to talk to legal, and we need to talk to IT security,
529
00:41:33,320 --> 00:41:39,880
we need to talk to ethics, and we probably need to hire a consultant and, you know, met
530
00:41:39,880 --> 00:41:46,600
with one of my colleagues and they're frustrated, but they're into two plus years of working
531
00:41:46,600 --> 00:41:50,800
on an AI governance framework for their organization.
532
00:41:50,800 --> 00:41:53,680
It's the most beautiful framework you will ever see.
533
00:41:53,680 --> 00:41:59,480
I hope they get it framed, but where we stand today after the tens of thousands of hours
534
00:41:59,480 --> 00:42:06,680
that have been invested into that thing is the organization has not deployed any AI solutions.
535
00:42:06,680 --> 00:42:12,600
So we're now in 2025 and they're not using AI after all that money.
536
00:42:12,600 --> 00:42:14,960
So I wouldn't get hung up.
537
00:42:14,960 --> 00:42:19,560
Yes, you need governance, but you need to be lean, smart, and action oriented of how you're
538
00:42:19,560 --> 00:42:21,640
going through that process.
539
00:42:21,640 --> 00:42:23,920
And yes, leadership needs to be engaged.
540
00:42:23,920 --> 00:42:28,920
We would have that as part of like our strategy framework or our diamondy.
541
00:42:28,920 --> 00:42:34,120
Yes, leadership needs to be aligned, but leadership doesn't need to lead and be the only decision
542
00:42:34,120 --> 00:42:36,680
maker in an innovation process.
543
00:42:36,680 --> 00:42:42,480
We need to keep our people and our end users engaged and part of that process as well.
544
00:42:42,480 --> 00:42:50,840
Yeah, I think hospitals need to have an innovation department which has control over a few beds
545
00:42:50,840 --> 00:42:59,280
in a marriage or in a floor and has full and has a budget and has the ability to deploy
546
00:42:59,280 --> 00:43:03,760
that budget without any oversight from leadership.
547
00:43:03,760 --> 00:43:11,600
I think there needs to be a distinct department which is separate from hospital governance,
548
00:43:11,600 --> 00:43:15,960
legal leadership that can, you know, incorporate these projects.
549
00:43:15,960 --> 00:43:16,960
Yeah, I completely agree.
550
00:43:16,960 --> 00:43:24,760
And I mean, like your audience is mostly in healthcare, so they will get this, but we
551
00:43:24,760 --> 00:43:27,040
see this in other areas as well.
552
00:43:27,040 --> 00:43:31,520
So I spent some time in academia as I mentioned.
553
00:43:31,520 --> 00:43:37,320
If we look at our org chart, how come we don't have a chief innovation officer or a chief
554
00:43:37,320 --> 00:43:38,480
technology officer?
555
00:43:38,480 --> 00:43:44,520
Like, isn't that strange that like some of these networks and systems are multi-million,
556
00:43:44,520 --> 00:43:49,320
sometimes billion dollar organizations and we don't have a chief innovation or chief
557
00:43:49,320 --> 00:43:51,000
technology officer?
558
00:43:51,000 --> 00:43:55,240
We also sometimes don't have a chief marketing officer or chief revenue officer either.
559
00:43:55,240 --> 00:43:59,040
And this goes for major academic health science networks.
560
00:43:59,040 --> 00:44:03,120
This goes for many of the universities and colleges in the country.
561
00:44:03,120 --> 00:44:08,920
So, you know, wearing my like business school hat of like, why do we find ourselves in the
562
00:44:08,920 --> 00:44:10,560
position that we are?
563
00:44:10,560 --> 00:44:15,720
Yeah, you know, we can tweak some things and we can blame economic policy or hate on whatever
564
00:44:15,720 --> 00:44:22,320
political party we want or individual, but structurally, we've made some really weird
565
00:44:22,320 --> 00:44:23,320
decisions, right?
566
00:44:23,320 --> 00:44:33,200
Like, if we want to see more innovation commercialized out of our universities and academic hospitals,
567
00:44:33,200 --> 00:44:38,360
how come there's not a chief commercialization officer at every university and hospital?
568
00:44:38,360 --> 00:44:44,600
If we want our hospitals to, you know, be global brands like the MIT, the Harford, the
569
00:44:44,600 --> 00:44:49,000
Stanford, the Oxford, it came from U of T and Ivy that have great brands.
570
00:44:49,000 --> 00:44:53,800
But how come we don't have a chief marketing and revenue officer at every university and
571
00:44:53,800 --> 00:44:54,800
hospital?
572
00:44:54,800 --> 00:45:01,240
So, there's things where structurally, we find ourselves where we are because we've made
573
00:45:01,240 --> 00:45:04,000
the decision, we've made the bed that we're lying in.
574
00:45:04,000 --> 00:45:07,800
And that's things that could change, you know, to your point, can you appoint someone?
575
00:45:07,800 --> 00:45:14,040
Can you give them a mandate money and people to go and change the way that care is delivered?
576
00:45:14,040 --> 00:45:15,040
Absolutely.
577
00:45:15,040 --> 00:45:19,480
There's nothing that's holding us back except our own ambition.
578
00:45:19,480 --> 00:45:21,080
Last question, Michael.
579
00:45:21,080 --> 00:45:26,760
If you could go back and talk to yourself 20 years ago, what advice would you give him?
580
00:45:26,760 --> 00:45:31,040
You have no idea what's coming, but take a breath, it's all going to be okay.
581
00:45:31,040 --> 00:45:32,040
Awesome.
582
00:45:32,040 --> 00:45:33,040
Thank you, Michael.
583
00:45:33,040 --> 00:45:34,560
Yeah, great to speak with you.
584
00:45:34,560 --> 00:45:41,560
Thank you.